Struggling to understand ordinal data? Often, it’s tricky to navigate this form of quantitative data that organizes information into ordered categories. This blog post simplifies the concept and provides clear examples of ordinal data in everyday scenarios.
We’ll delve into its collection methods, and analysis techniques and share valuable tips for exploiting its potential.
Key Takeaways
- Ordinal data is a type of quantitative data that ranks or orders categories, but the differences between the categories may not be equal.
- Examples of ordinal data include interest level, education level, socio-economic status, and satisfaction level.
- Collecting ordinal data involves choosing the appropriate level of measurement and using tools like Likert scales.
- Analyzing ordinal data requires descriptive statistics to summarize the data and statistical tests to compare groups.
Understanding Ordinal Data
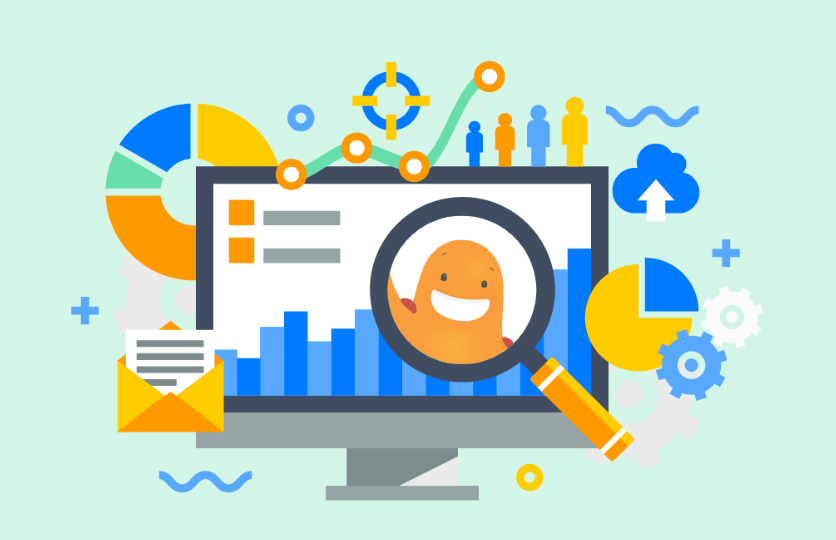
Ordinal data is a type of variable that can be ranked or ordered, and it is important to understand its levels of measurement and characteristics.
Levels of measurement
Data can be measured in four ways. One way is ordinal data. The three other ways are nominal, interval, and ratio data. Ordinal data groups things into an order or rank. Nominal data groups things into categories with no set order.
Interval and ratio types of data have a set amount between each group.
Characteristics of ordinal data
Ordinal data is one type. It gets put into groups or ranks that have an order to them. For example, there are ranks like ‘first’, ‘second’, and ‘third’. The steps in the rank hold a clear place.
This means you know which is more and which is less. But, each step does not have the same size from one to the next one. So, with ordinal data we can’t tell how much different each step is from another.
You might also see ordinal data on a scale or list of options like “very sad,” “sad,” “okay,” “happy,” and “very happy”. We only use some math works with this type of data like finding out what comes up most often or what stands in the middle of all answers given but not adding up or timesing together these items’ values.
Comparison to other types of variables
Ordinal data is unique and different from other types of variables in several key ways. Here’s a comparison between ordinal data and nominal, interval, and ratio data.
Type of Data | Definition | Characteristics | Examples |
---|---|---|---|
Ordinal Data | Quantitative data that is categorized into ordered categories or ranks. | Categories have a natural order, but differences between categories are not equal. Mathematical operations like addition or multiplication are not meaningful. | Ratings, rankings, Likert scales. |
Nominal Data | Data that is categorized into unordered groups or categories. | Categories have no order or scale. Only mathematical operation possible is counting. | Gender, marital status, types of food. |
Interval Data | Numeric data with consistent intervals between each point. | Differences between data points are meaningful. Addition and subtraction are possible, but multiplication and division are not. | Temperature measured in Celsius or Fahrenheit, IQ scores. |
Ratio Data | Numeric data with a consistent interval and a true zero point. | All mathematical operations are possible. Ratio comparisons are meaningful. | Height, weight, age, income. |
As can be seen from the table, the key difference between ordinal data and other types is the presence of a natural order in the categories and the inability to perform addition or multiplication on the data. This unique nature of ordinal data makes it valuable in specific types of analyses and research.
Examples of Ordinal Data
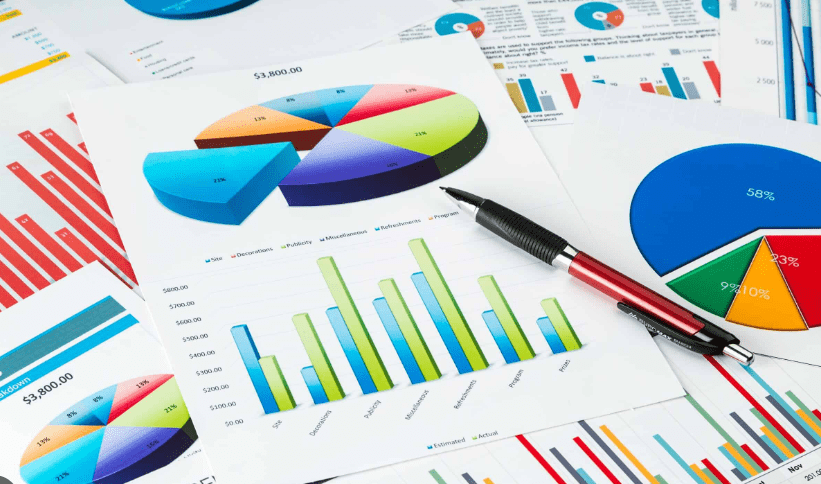
Interest level, education level, socio-economic status, satisfaction level, and comparison are some examples of ordinal data.
Interest level
Interest level is an example of ordinal data. It measures the degree of interest someone has in a particular topic or activity. For example, on a scale from 1 to 5, someone might rate their interest in technology as a 3.
In ordinal data, the categories have a natural order or rank, but the differences between them may not be equal. So, while we can say that someone rates their interest higher than another person’s rating, we cannot determine by how much more they are interested.
This type of data is commonly collected through surveys or questionnaires and can be analyzed using descriptive statistics like frequency distribution and measures of central tendency such as median or mode.
Education level
Education level is an example of ordinal data. It represents the ranking or order of someone’s educational achievement, such as high school diploma, bachelor’s degree, or master’s degree.
In ordinal data like education level, there is a clear progression from one category to another, but the differences between them are not necessarily equal. For example, the difference between having a high school diploma and a bachelor’s degree is not the same as the difference between having a bachelor’s degree and a master’s degree.
Understanding education level as ordinal data helps researchers analyze and interpret its impact on various outcomes like job opportunities or income levels accurately.
Socio-economic status
Socio-economic status, or SES, is a type of ordinal data that measures a person’s position in society based on factors like their income, education level, and occupation. It helps researchers understand how social and economic factors can impact people’s lives.
For example, someone with a high SES may have more access to opportunities and resources compared to someone with a low SES. Collecting data on socio-economic status can be done through surveys or questionnaires that ask participants about their income range, educational attainment, and job title.
Analyzing this data allows researchers to identify patterns and trends related to social inequality and inform policies aimed at reducing disparities in society.
Satisfaction level
Satisfaction level is an example of ordinal data. It measures people’s satisfaction or contentment with a product, service, or experience. In this case, the categories are ranked from low to high satisfaction levels.
For example, in a customer survey, participants might rate their satisfaction on a scale from 1 to 5 or use words like “very dissatisfied,” “dissatisfied,” “neutral,” “satisfied,” and “very satisfied.” The important thing to remember about ordinal data is that the categories have a rank order but the differences between them may not be equal.
Analyzing satisfaction levels involves calculating summary statistics like frequency distribution and finding the mode (the most common level of satisfaction) or median (the middle value).
Comparison
When it comes to ordinal data, it’s crucial to understand how it compares to other types of data categories, specifically nominal, interval, and ratio data. This comparison is particularly important because it can influence the manner in which you gather, analyze, and interpret your data.
Type of Data | Definition | Examples | Key Characteristics |
---|---|---|---|
Ordinal | Quantitative data that is categorized in an ordered sequence. | Rankings, ratings, and Likert scales | The categories have a natural order, but the differences between the categories may not be equal. |
Nominal | Qualitative data that is categorized without any order or priority. | Gender, hair color, and nationality | The categories can be named but not ranked or ordered. |
Interval | Quantitative data with continuous values where the difference between values is meaningful. | Temperature in degrees Celsius, IQ scores, and SAT scores | The intervals between the categories are equal, but there is no true zero point. |
Ratio | Quantitative data with continuous values where both the difference between values and the ratio of values are meaningful. | Age, height, weight, and income | There is a true zero point, and both subtraction and division are meaningful. |
Understanding these differences helps to make a more informed choice when deciding which data type is best suited for your specific research purposes.
Collecting Ordinal Data
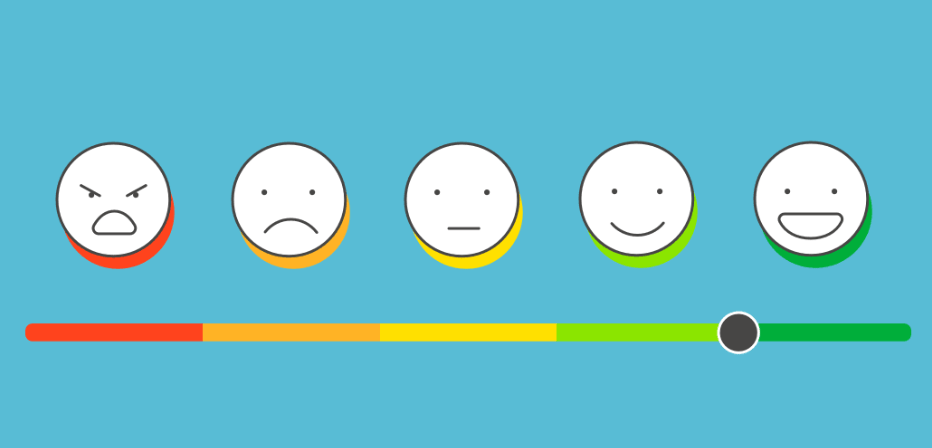
To collect ordinal data, researchers must choose the appropriate level of measurement and utilize tools such as Likert scales to gather information on ranked categories.
Choosing the level of measurement
Ordinal data is a type of quantitative data that falls under one of the four levels of measurement. When selecting the level of measurement for your data, it is important to consider the nature of your variables.
Ordinal data is suitable when you have categories that can be ranked in a particular order, such as rankings or ratings. It is different from nominal data, where categories are simply labeled without any inherent order.
By choosing ordinal measurement, you can capture the natural rank order and understand relationships between categories based on their position in the scale. This allows for easier analysis and interpretation of research findings later on.
Using Likert scales
Likert scales are a popular method for collecting ordinal data. Here are some key points to know about using Likert scales:
- Likert scales involve presenting respondents with a statement or question and asking them to indicate their level of agreement or disagreement.
- The response options typically range from strongly agree to strongly disagree, with a neutral option in the middle.
- Each response option is assigned a numerical value, allowing for quantitative analysis of the data.
- Likert scales can be used in surveys, questionnaires, or any research instrument where opinions or attitudes need to be measured.
- They are commonly used in areas such as customer satisfaction surveys, employee feedback surveys, and market research studies.
- When using Likert scales, it’s important to ensure clear and concise wording of the statements or questions.
- The number of response options should also be carefully considered. Too many options can lead to respondent confusion, while too few options may not capture the full range of opinions.
- Data collected using Likert scales can be analyzed using various descriptive statistics such as mean, median, and mode.
- Statistical tests like chi – square test and Mann–Whitney U test can also be applied when comparing groups based on their responses.
Analyzing Ordinal Data
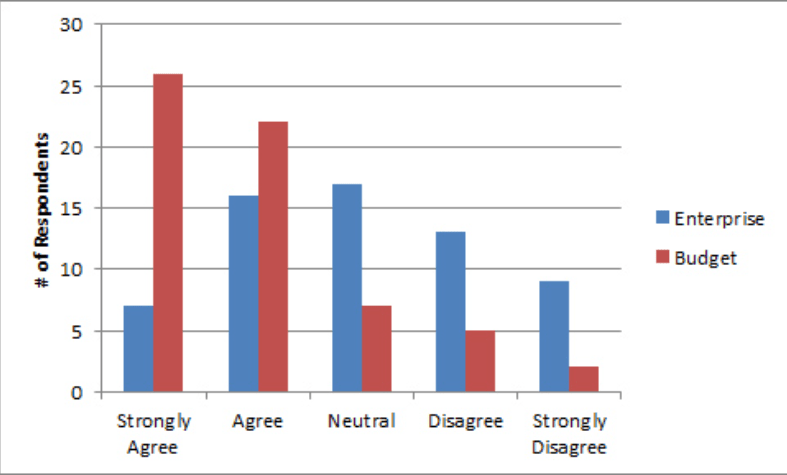
Analyzing Ordinal Data involves using descriptive statistics to summarize the data, such as measures of central tendency and variability, as well as conducting statistical tests to determine if there are significant differences between groups.
Descriptive statistics
Descriptive statistics is an important part of analyzing ordinal data. It helps us understand the characteristics and patterns in the data. With descriptive statistics, we can calculate summary measures such as frequency distribution, median, and mode.
These measures give us a clear picture of how the categories are distributed and what values are most common. For example, if we have ordinal data on satisfaction levels of customers, descriptive statistics can tell us the most frequent level of satisfaction and the overall pattern of responses.
Additionally, visualization techniques like bar charts or stacked bar charts can help us represent this information in a visual format that is easy to understand. This allows us to spot trends or differences between different categories quickly.
Remember, while descriptive statistics provide valuable insights into ordinal data, it’s important to note that mathematical operations like addition or multiplication are not meaningful with this type of data.
Measures of central tendency
Ordinal data provides some important measures of central tendency that can help in data interpretation. These include the mode, median, and sometimes, the mean. However, the mean is often not a trustworthy measure for ordinal data due to the inherent inequality between the categories.
Measure | Description | Application to Ordinal Data |
---|---|---|
Mode | Most frequently occurring value in the data set | Can be used with ordinal data to identify the most commonly occurring category or rank |
Median | Middle value in a sorted data set | Can be used with ordinal data to find the middle ranking or category, assuming the data has a clear order |
Mean | Arithmetic average of the data set | Less frequently used with ordinal data due to the unequal distances between categories. However, may still provide a rough estimate of the central tendency if required |
These are key statistics used for ordinal data analysis. They provide a simple way to summarize complex or large volumes of data into a single value. As ordinal data often involves rankings or ratings, these measures can help to identify any common trends or patterns in the responses.
Variability
Variability is an important aspect when analyzing ordinal data. It refers to the amount of dispersion or spread within the ranked categories. In other words, it measures how much the values in a dataset differ from each other.
While descriptive statistics like frequency distribution and measures of central tendency (such as median and mode) give us information about the average or typical value, variability provides insights into the range or diversity of values.
By understanding variability in ordinal data, we can gain a better understanding of the patterns and differences that exist within our dataset and make more informed decisions based on these findings.
Statistical tests
Statistical tests are used to analyze ordinal data and draw conclusions from the results. Here are some important things to know about statistical tests for ordinal data:
Statistical Test | Description |
---|---|
Non-parametric tests | Due to the characteristics of ordinal data, non-parametric tests are often used. These tests do not assume a specific distribution of the data. |
Mann-Whitney U test | This test compares two groups and determines if there is a significant difference between them based on their ranks. |
Kruskal-Wallis test | This test is used when comparing more than two groups to see if there is a significant difference in their rankings. |
Wilcoxon signed-rank test | This test is used when comparing related samples, such as before and after measurements, to determine if there is a significant difference in their ranks. |
Chi-square test for independence | This test assesses whether there is a relationship between two categorical variables measured on an ordinal scale. |
Spearman’s rank correlation coefficient | This statistical measure determines the strength and direction of the relationship between two ordinal variables. |
Limitations and Applications of Ordinal Data
Ordinal data has its limitations, primarily because it lacks precise measurements and fixed intervals. However, it finds extensive applications in surveys, research studies, customer service evaluations, and job applications where subjective ranking or ordering is necessary for analysis and decision-making processes.
QuestionPro’s advanced survey software can aid in effectively managing ordinal data collection and analysis while providing valuable insights into the respondents’ preferences and opinions.
Uses in surveys, research, customer service, and job applications
Ordinal data is widely used in surveys, research, customer service, and job applications. It provides valuable insights into people’s preferences, opinions, and rankings. Here are some specific ways in which ordinal data is utilized in these areas:
Use | Description |
---|---|
Surveys | Ordinal data allows researchers to gather information on customer satisfaction levels, product preferences, and opinions on various topics. This data helps businesses make informed decisions and improve their offerings. |
Research | In academic studies or scientific research, ordinal data enables researchers to measure attitudes, behavior patterns, and performance rankings. This data is essential for drawing conclusions and making comparisons between different groups. |
Customer Service | Many customer satisfaction surveys utilize ordinal scales to gather feedback on the quality of products or services provided. Companies can use this information to identify areas for improvement and enhance customer experiences. |
Job Applications | Ordinal data is often used in job applications to assess candidates’ qualifications relative to each other. For example, a questionnaire may ask applicants to rank their proficiency levels in specific skills or rate their comfort level with certain tasks. |
How QuestionPro can help with managing ordinal data
QuestionPro is a powerful survey platform that can assist in managing and analyzing ordinal data. It provides features like Likert scale questions, which are commonly used to collect ordinal data.
With QuestionPro, you can easily design surveys and questionnaires with ranked response options to gather the necessary information. Additionally, it offers robust data analysis tools that allow you to generate descriptive statistics for your ordinal data, such as frequency distribution, median, and mode.
This helps in understanding the distribution and central tendency of your data. Furthermore, QuestionPro enables you to visualize your ordinal data through bar charts or stacked bar charts for easy interpretation.
Tips for gaining insights with ordinal data
Tip | Description |
---|---|
Use Appropriate Statistical Tests | When analyzing ordinal data, it’s important to use statistical tests that are suitable for ranked categories. Tests like the Mann-Whitney U test or Spearman’s rank correlation can provide valuable insights. |
Avoid Treating Ordinal Data as Continuous | Remember that ordinal data does not have equal intervals between categories. Avoid making assumptions about the magnitude of differences between the categories and refrain from using mathematical operations like addition or multiplication. |
Visualize the Data Effectively | Use bar charts or stacked bar charts to visually represent ordinal data. This can help in understanding patterns, comparisons, and trends within the ranked categories. |
Take into Account Context and Meaning | Consider the context of the ordinal data and its implications. Understand how each category relates to the research question or objective, and interpret the results accordingly. |
Consider Combining with Other Types of Data | Sometimes, combining ordinal data with other types of variables, such as nominal or ratio variables, can provide a more comprehensive analysis and deeper insights. |
Remember, by following these tips when working with ordinal data, you can gain meaningful insights and make informed decisions based on your analysis.
Frequently asked questions
- What is ordinal data?
- How is ordinal data different from other types of data?
- What are some examples of ordinal data?
- What methods can be used to collect ordinal data?
- How can I analyze and interpret ordinal data?
- Can ordinal data be converted into numerical values for analysis?
- Is it possible to perform mathematical operations on ordinal data?
- How can ordinal data be presented visually?
- What are the limitations of using ordinal data in research or analysis?
- How can QuestionPro help with managing and analyzing ordinal data?
Conclusion
In conclusion, understanding ordinal data is important for collecting and analyzing information in a meaningful way. Ordinal data is a type of quantitative data that is organized into ordered categories or ranks.
It differs from nominal and interval/ratio data because the categories have a natural order or scale, but the differences between them may not be equal. Examples of ordinal data include rankings, ratings, and Likert scales.
While mathematical operations like addition or multiplication are not meaningful with ordinal data, it still provides valuable insights and can be used in statistical analyses and modeling.
Frequently Asked Questions
What is ordinal data?
Ordinal data is a type of categorical data that represents information with a specific order or ranking, such as rating scales or survey responses with options like “low,” “medium,” and “high.”
What are some examples of ordinal data?
Examples of ordinal data include rankings in sports competitions, educational grades (A, B, C), customer satisfaction ratings (very satisfied, satisfied, dissatisfied), or levels of agreement/disagreement (strongly agree, agree, neutral, disagree).
How is ordinal data collected?
Ordinal data can be collected through surveys or questionnaires where respondents provide their rank-order preferences or opinions by selecting from a predefined set of options.
Can I analyze ordinal data using statistical methods?
Yes! Ordinal data can be analyzed using various statistical methods like the Mann-Whitney U test or Spearman’s rank correlation coefficient to examine relationships or differences between variables.
Is it possible to convert ordinal data into numerical values for analysis?
While it may be tempting to assign numerical values (e.g., 1 for low and 5 for high) to represent ranks in ordinal data during analysis, caution should be exercised as these numbers do not reflect equal distances between categories and may mislead interpretation.
Author
-
Anisha Jain, a dynamic professional in the sports SaaS industry, transitioned from economics to digital marketing, driven by her passion for content writing. Her tenure at TBC Consulting culminated in her role as CEO, where she honed her skills in digital strategy, branding, copywriting, and team management. Anisha's expertise encompasses various aspects of digital marketing, including 360-degree marketing, digital growth consulting, client communication, and business development, making her a versatile asset in the SaaS domain.
View all posts