Data drives analytics, decision-making, and automation across industries. It exists in various forms, with continuous and discrete data being fundamental types. Understanding these is crucial for data analysis, AI, and machine learning.
This guide explores their differences, real-world applications, and emerging trends in technology, business, and science.
Key Takeaways
- Continuous data is measured and can take any value within a range, while discrete data is countable with distinct values.
- Continuous data powers real-time monitoring and AI-driven decision-making.
- Discrete data supports event tracking, business analytics, and classification models.
- AI, IoT, and blockchain are driving the need for efficient data processing.
- Hybrid models integrating both data types enhance analytics, automation, and decision-making across industries.
What is Continuous Data?
Continuous data consists of measurable values that can take any number within a range, including decimals or fractions. Unlike discrete data with fixed values, continuous data allows infinite precision.
It’s commonly used for variables that change smoothly over time, such as temperature, speed, or body measurements, making it essential in fields like science, engineering, healthcare, and finance.
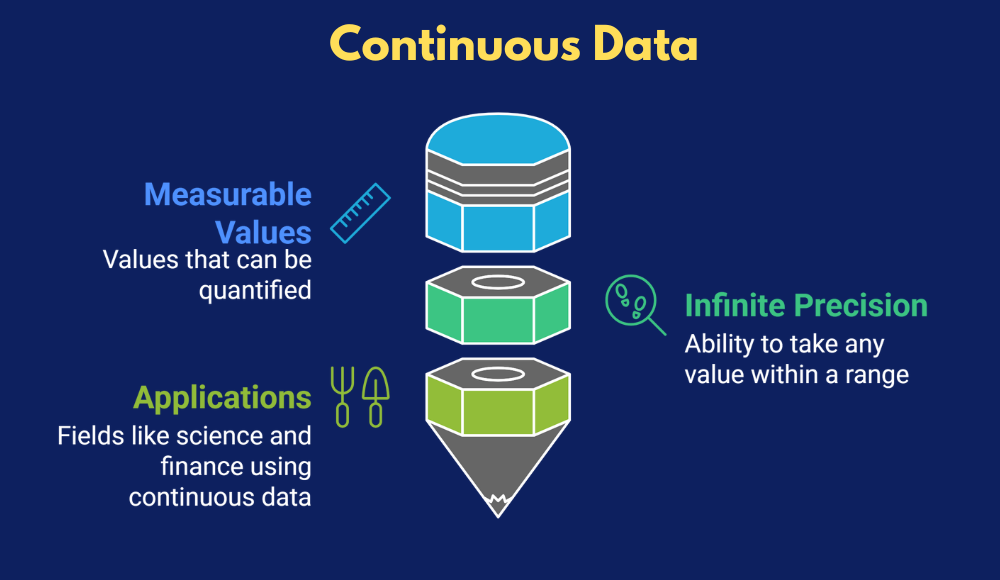
Characteristics of Continuous Data
- Can take any value within a given range.
- Measured rather than counted.
- Includes decimals and fractions for greater accuracy.
- Often represented by line graphs, histograms, and probability distributions.
Examples of Continuous Data Across Different Fields
This table provides examples of continuous data along with how they are measured across various fields.
Category | Example | How It’s Measured |
---|---|---|
Physical Measurements | Height and weight of a person | Measured in centimeters, kilograms, pounds |
Temperature readings | Measured in Celsius, Fahrenheit, Kelvin | |
Speed of a moving vehicle | Measured in kilometers per hour (km/h), mph | |
Time and Finance | Time duration (work hours, exercise time) | Measured in hours, minutes, seconds |
Stock market prices | Measured in currency values with decimals | |
Inflation rate | Measured as a percentage over time | |
Science and Medicine | Heart rate variability | Measured in beats per minute (bpm) |
Blood pressure | Measured in mmHg (millimeters of mercury) | |
Oxygen saturation levels | Measured in percentage (%) | |
Engineering and Technology | Sound levels | Measured in decibels (dB) |
Machine temperature | Measured in degrees (°C, °F) | |
Battery charge level in devices | Measured in percentage (%) | |
Environmental Data | Rainfall measurements | Measured in millimeters (mm) |
Wind speed | Measured in kilometers per hour (km/h) | |
River water levels | Measured in meters (m) |
Latest Trends in Continuous Data
Continuous data is playing a significant role in emerging technologies, helping industries make real-time decisions and automate processes.
AI and Machine Learning for Predictive Analytics
Machine learning models depend on continuous data to detect trends, predict future events, and automate decision-making. AI algorithms analyze large amounts of real-time continuous data to make accurate predictions.
- AI-powered health monitoring systems analyze heart rate variability, oxygen levels, and blood pressure readings to detect early signs of medical conditions.
- Stock market prediction models use historical price movements, trading volume, and real-time financial data to forecast future trends.
- AI-based weather forecasting systems analyze temperature, humidity, and wind speed to provide accurate climate predictions.
IoT and Real-Time Data Streaming
Continuous data is widely used in Internet of Things (IoT) devices, enabling smart technology to function efficiently. These devices collect, process, and transmit data in real time for automation and control systems.
- Smartwatches and fitness trackers monitor step count, heart rate, and calories burned continuously to provide health insights.
- Self-driving cars analyze continuous data from sensors, including speed, acceleration, road conditions, and pedestrian movements to make real-time driving decisions.
- Industrial automation systems track machine temperature, pressure levels, and vibration patterns to detect malfunctions and prevent failures.
Edge Computing for Faster Data Processing
Traditionally, continuous data was sent to cloud-based servers for processing. However, edge computing now allows data to be processed locally on devices, reducing latency and improving response times.
- Security surveillance cameras use edge computing to analyze live video streams, identifying security threats in real time.
- Healthcare wearable devices process heart rate and temperature data on-device, allowing for instant alerts on abnormalities.
- Manufacturing systems analyze sensor data locally, detecting defects without needing to send large amounts of data to centralized cloud storage.
What is Discrete Data?
Discrete data consists of distinct, countable values that cannot be divided into smaller parts. It represents whole numbers, such as employee count, defective products, or survey responses.
Unlike continuous data, it is finite and often visualized using bar charts, pie charts, or frequency tables. Discrete data helps in statistical analysis, making it valuable for business metrics, quality control, and customer insights. It is widely used in decision-making processes across industries.
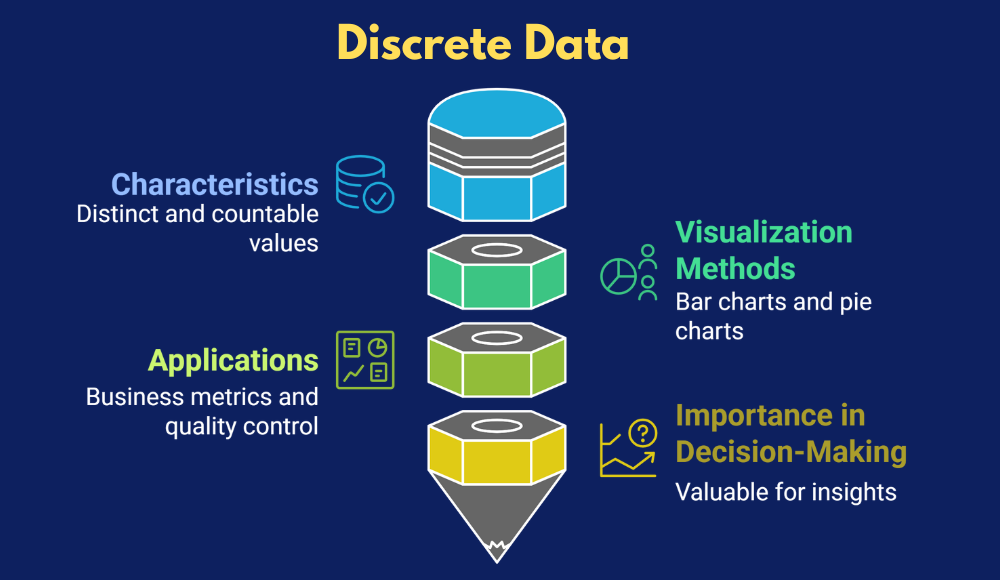
Characteristics of Discrete Data
- Consists of separate, distinct values.
- Always counted, not measured.
- Cannot take decimal or fractional values.
- Typically represented using bar charts or pie charts.
Examples of Discrete Data Across Different Fields
This table highlights examples of discrete data and how they are measured in various domains.
Category | Example | How It’s Measured |
---|---|---|
Business & Finance | Number of employees in a company | Counted as whole numbers |
Sales transactions per day | Counted as individual purchases | |
Education | Number of students in a class | Counted as whole numbers |
Exam scores (out of 100) | Counted as discrete points | |
Healthcare | Number of patients in a hospital | Counted as whole numbers |
Number of doctor visits per year | Counted as individual visits | |
Technology | Number of devices connected to a network | Counted as individual connections |
Software bugs reported in a month | Counted as distinct issues | |
Sports & Entertainment | Goals scored in a match | Counted as whole numbers |
Number of tickets sold | Counted as individual sales | |
Manufacturing | Defective products in a batch | Counted as separate units |
Number of items produced | Counted as whole numbers |
Latest Trends in Discrete Data
Discrete data is crucial for decision-making in business intelligence, predictive modeling, and automation.
As technology advances, industries are leveraging discrete data for analytics, forecasting, and optimization. It helps organizations track specific events, measure key performance indicators, and make data-driven decisions efficiently.
AI and Data Analytics for Trend Detection
Machine learning models use discrete data to identify patterns and trends in various industries:
- Retail businesses analyze the number of daily transactions to optimize inventory and marketing strategies, ensuring that supply meets customer demand.
- Educational platforms track quiz scores and student attendance to personalize learning experiences, improving engagement and retention.
- Social media platforms analyze discrete engagement metrics (likes, shares, comments) to improve content recommendations, increasing user interaction and time spent on platforms.
By leveraging discrete data, businesses can fine-tune their operations, enhance user experiences, and create more targeted strategies.
IoT and Smart Data Collection
With the rise of the Internet of Things (IoT), discrete data collection is becoming more automated:
- Smart home systems track the number of times a door is opened to enhance security, providing homeowners with insights into activity patterns.
- Industrial sensors count machine cycles to schedule predictive maintenance, reducing downtime and increasing equipment lifespan.
- Transportation systems monitor vehicle counts at toll booths for traffic management, helping city planners optimize road usage and reduce congestion.
These applications enable real-time monitoring, automated responses, and improved resource allocation based on precise event tracking.
Automation and AI Decision-Making
AI-driven automation relies on discrete data to improve efficiency:
- Chatbots analyze the number of customer queries to adjust response models, ensuring faster and more accurate support.
- Logistics companies track package counts to streamline deliveries, optimizing routes and improving last-mile efficiency.
- Manufacturing plants optimize production schedules based on completed unit counts, reducing waste and maximizing productivity.
By integrating discrete data into AI-driven workflows, companies can enhance decision-making, improve customer satisfaction, and boost overall operational performance.
Discrete vs. Continuous Data Key Differences
Discrete data consists of countable, distinct values, while continuous data includes measurable values within a range. Knowing the difference improves data analysis and decision-making.
The table below highlights the key differences between discrete and continuous data, helping to understand their characteristics and applications.
Feature | Discrete Data | Continuous Data |
---|---|---|
Nature | Countable, distinct values | Measurable, can take any value |
Includes | Whole numbers only | Can include decimals & fractions |
Examples | Number of students, product defects, sales transactions | Temperature, weight, speed, stock prices |
Visualization | Bar charts, pie charts, frequency tables | Line graphs, histograms, probability distributions |
Real-World Applications of Continuous and Discrete Data
Continuous and discrete data play a crucial role across industries, helping businesses, healthcare providers, and manufacturers make data-driven decisions. Below are key applications of both data types in different sectors.
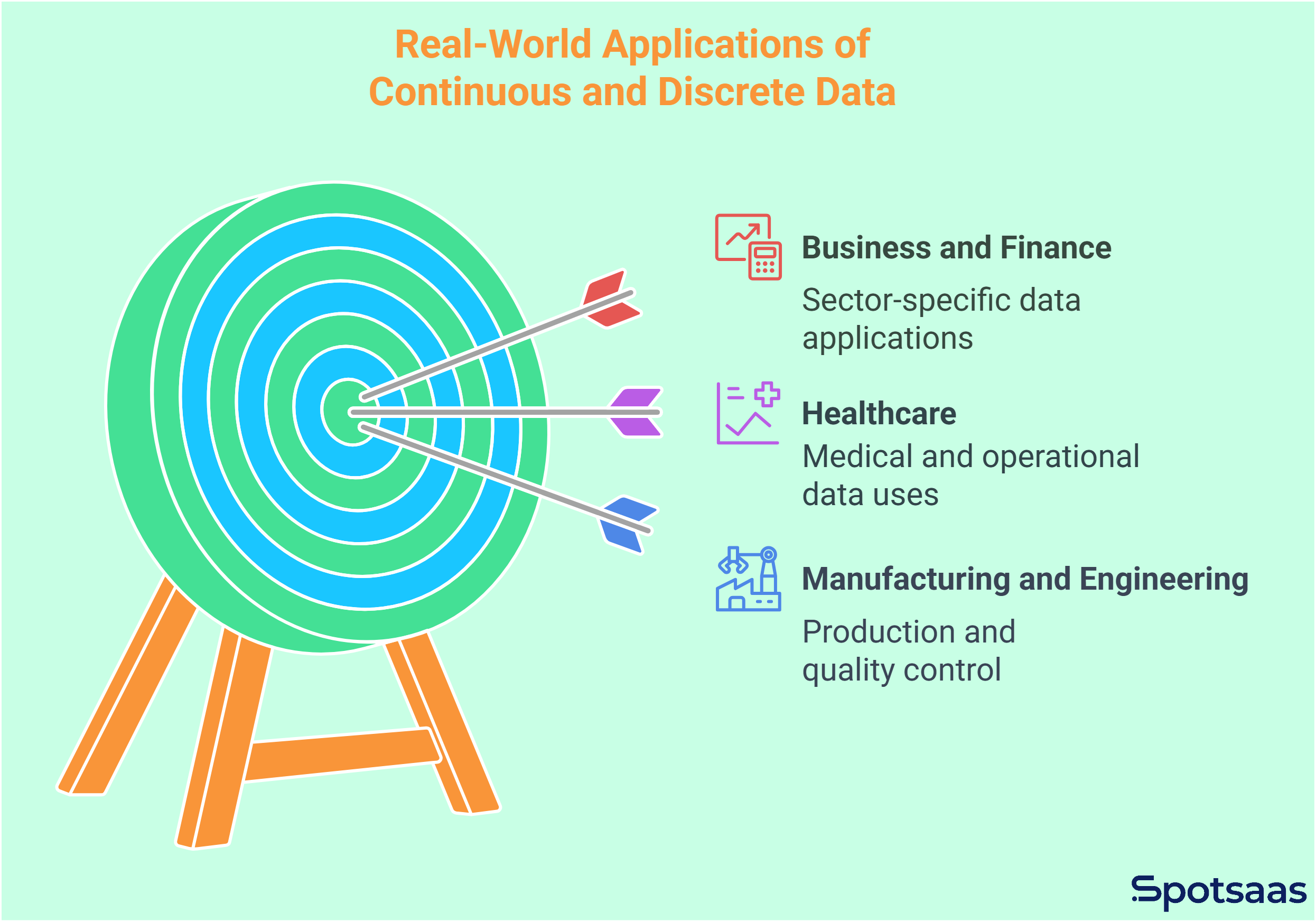
Business and Finance
Continuous Data in Business and Finance
Continuous data is essential for tracking financial trends and making informed business decisions.
- Stock prices fluctuate throughout the trading day, requiring real-time monitoring for trend analysis and investment decisions.
- Revenue trends help businesses forecast earnings, optimize pricing, and assess financial stability.
Discrete Data in Business and Finance
Discrete data allows businesses to measure specific events and track key performance indicators.
- Number of customer transactions helps analyze sales trends, customer behavior, and seasonal demand.
- Product purchases provide insights into consumer preferences and inventory management.
Healthcare
Continuous Data in Healthcare
Continuous data is vital for patient monitoring and medical research, enabling real-time analysis and early detection.
- Blood pressure levels are monitored continuously in hypertensive patients to adjust medications and prevent complications.
- Heart rate monitoring helps diagnose arrhythmias and assess cardiovascular health.
Discrete Data in Healthcare
Discrete data is used for hospital operations, patient statistics, and healthcare trend analysis.
- Number of patients admitted helps hospitals allocate resources, plan staffing, and manage patient care efficiently.
- Count of medicines prescribed allows healthcare providers to monitor medication usage and detect treatment trends.
Manufacturing and Engineering
Continuous Data in Manufacturing and Engineering
Continuous data helps maintain quality control and optimize production efficiency.
- Machine speed is tracked to prevent mechanical failures and ensure smooth operations.
- Product dimensions are measured continuously to detect defects and maintain quality standards.
Discrete Data in Manufacturing and Engineering
Discrete data is used to measure production efficiency and improve quality assurance.
- Number of defective items in a batch helps identify manufacturing issues and implement corrective actions.
- Total units produced enables manufacturers to track productivity, manage supply chains, and forecast production needs.
By leveraging both continuous and discrete data, industries enhance decision-making, improve efficiency, and optimize operational processes.
How to Analyze Continuous vs Discrete Data
Analyzing continuous and discrete data requires different collection methods, statistical approaches, and solutions to potential challenges. Proper analysis ensures accuracy in decision-making, trend forecasting, and data-driven insights across industries.
Data Collection Methods
Continuous data collection
Continuous data is measured rather than counted and is typically collected using instruments, sensors, and automated systems that provide high-precision readings.
- Sensors are used in industries to monitor environmental conditions such as temperature, humidity, and pressure in real time.
- Meters measure continuous variables like electricity usage, fuel consumption, and vehicle speed.
- Digital logs track continuous metrics such as stock price movements, heart rate fluctuations, and climate patterns.
- Time-series recordings capture continuous changes in variables over time, such as monitoring machine performance or rainfall accumulation.
Continuous data collection is essential in industries requiring real-time decision-making, predictive modeling, and monitoring dynamic conditions.
Discrete data collection
Discrete data is countable and is collected through event tracking, surveys, and manual recording methods.
- Surveys gather numerical and categorical discrete data, such as customer feedback, product ratings, and demographic information.
- Event logs record user actions, such as website visits, app downloads, and transactions.
- Tally counts track fixed values such as the number of units sold, defective products in manufacturing, or attendees at an event.
- Checklists are used in audits and inspections to track compliance, inventory levels, and production cycle counts.
Discrete data collection is widely used in business intelligence, statistical reporting, and decision-making that involves categorization and counting.
Statistical Techniques
Continuous data analysis
Continuous data analysis involves statistical methods that measure trends, relationships, and variations.
- Regression models are used to predict future values based on observed trends, such as estimating sales growth based on historical revenue.
- Standard deviation measures the spread of data points, helping in risk assessment and quality control.
- Probability distributions (normal, exponential, and uniform distributions) describe the likelihood of different continuous values occurring, which is useful in financial modeling and machine learning.
- Moving averages help smooth out short-term fluctuations to identify long-term trends in time-series data.
Continuous data analysis is crucial for industries where precision, forecasting, and optimization play a key role.
Discrete data analysis
Discrete data is analyzed using methods that count occurrences, classify categories, and calculate probabilities.
- Frequency distribution represents how often different discrete values appear, such as customer orders per day or defect rates in production.
- Classification models in machine learning categorize data into distinct groups, such as fraud detection in banking transactions.
- Probability analysis estimates the likelihood of events, such as predicting the number of customer visits on a weekend.
- Chi-square tests help determine relationships between categorical variables, such as analyzing customer preferences based on age groups.
Discrete data analysis is commonly applied in business analytics, market research, and risk assessment.
Future Trends in Continuous and Discrete Data
Technology is transforming how continuous and discrete data are collected, analyzed, and applied. Businesses, healthcare, finance, and manufacturing are leveraging AI, IoT, and edge computing to improve efficiency, decision-making, and automation.
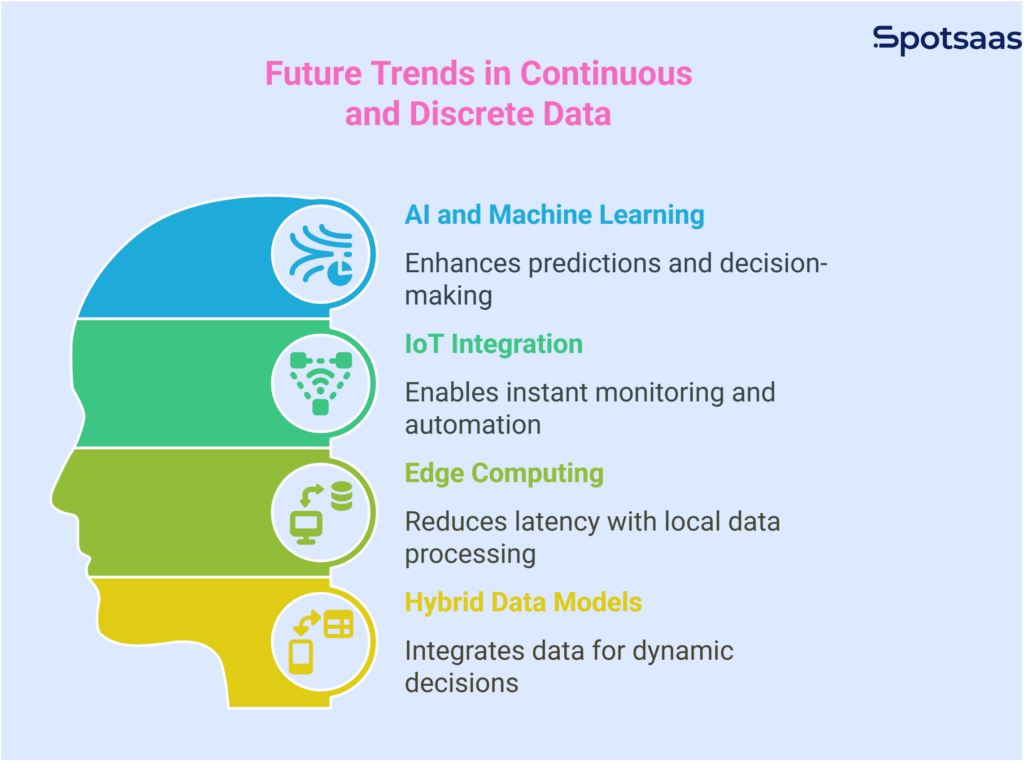
AI and Machine Learning for Data Analysis
AI uses continuous data for real-time forecasting in finance, healthcare, and automation, while discrete data enhances classification, fraud detection, and recommendations.
Integrating both enables more accurate predictions and smarter decision-making across industries. As AI advances, the ability to process and combine these data types will further enhance automation, efficiency, and adaptive learning in various applications.
Real-Time Data Processing and IoT Integration
IoT enables instant monitoring and automation, improving decision-making. Continuous data optimizes smart homes, self-driving cars, and manufacturing, while discrete data powers event-driven analytics in website tracking, cybersecurity, and supply chain management.
As IoT adoption grows, real-time data processing will become even more crucial for efficiency and automation.
Edge Computing and Decentralized Processing
Edge computing reduces latency by processing data locally. Continuous data improves IoT efficiency in industrial monitoring, healthcare wearables, and autonomous systems.
Discrete data enhances security in blockchain transactions, identity verification, and smart contract automation. With the rise of decentralized computing, businesses are leveraging local processing to reduce reliance on centralized cloud systems.
Hybrid Data Models for Analytics
Integrating continuous and discrete data improves decision-making. Retailers use both for personalized marketing, healthcare AI enhances diagnostics, and financial firms refine investment strategies, driving automation and accuracy across industries.
The combination of these data types enables businesses to make more dynamic, data-driven decisions in real-time.
Conclusion
Understanding continuous and discrete data is key for effective analysis, AI, and decision-making. Continuous data supports real-time monitoring and forecasting, while discrete data aids in event tracking and classification.
As AI, IoT, and blockchain evolve, integrating both data types enhances analytics, automation, and business intelligence, enabling smarter, data-driven decisions.
Hybrid data models are transforming industries, optimizing efficiency, and improving predictive capabilities for better strategic planning. Businesses that effectively leverage both data types gain a competitive edge in an increasingly digital world.
Frequently Asked Questions
What is the difference between continuous and discrete data?
Continuous data is measurable and can take any value within a range, while discrete data consists of countable, distinct values with no intermediate points.
How is continuous data used in AI and machine learning?
Continuous data powers real-time forecasting, predictive analytics, and automation in areas like finance, healthcare, and industrial operations.
Why is discrete data important in analytics?
Discrete data helps in classification, event tracking, and business analytics, supporting AI-driven recommendations, fraud detection, and structured decision-making.
Can continuous and discrete data be used together?
Yes, hybrid models combine both data types to improve predictions and decision-making, such as in airline pricing systems or personalized marketing.
How is IoT transforming continuous and discrete data usage?
IoT devices collect continuous data for real-time monitoring (e.g., temperature sensors) and discrete data for event-based tracking (e.g., counting login attempts).
Author
-
Suma Bavigadda, a dynamic professional in the digital space, transitioned from economics to content marketing, fueled by her passion for writing. With a strong foundation in SEO and content strategy, she has honed her skills in crafting compelling content, optimizing search performance, and driving digital growth. Her expertise spans content creation, SEO optimization, branding, and audience engagement, making her a valuable asset in the ever-evolving digital landscape.
View all posts